13 Nov 2017
|
batch
image
tensorflow
CelebA Image batch로…
%matplotlib inline
import matplotlib.pyplot as plt
import numpy as np
import imageio
import tensorflow as tf
import os
os.environ["CUDA_DEVICE_ORDER"]="PCI_BUS_ID"
os.environ["CUDA_VISIBLE_DEVICES"]="7"
def batch(file_list, batch_size=16):
fileQ = tf.train.string_input_producer(file_list, shuffle=False)
reader = tf.WholeFileReader()
filename, data = reader.read(fileQ)
image = tf.image.decode_jpeg(data, channels=3)
img = imageio.imread(file_list[0])
w, h, c = img.shape
shape = [w, h, 3]
image.set_shape(shape)
min_after_dequeue = 10000
capacity = min_after_dequeue + 3 * batch_size
queue = tf.train.shuffle_batch(
[image], batch_size=batch_size,
num_threads=4, capacity=capacity,
min_after_dequeue=min_after_dequeue, name='synthetic_inputs')
crop_queue = tf.image.crop_to_bounding_box(queue, 50, 25, 128, 128)
resized = tf.image.resize_nearest_neighbor(crop_queue, [64, 64])
return tf.to_float(resized)
import os
dir_ = '/root/CelebA/images'
jpgs = os.listdir(dir_)
filelist = [os.path.join(dir_, jpg) for jpg in jpgs]
plt.imshow(imageio.imread(filelist[0]))
<matplotlib.image.AxesImage at 0x7f95ae746d68>
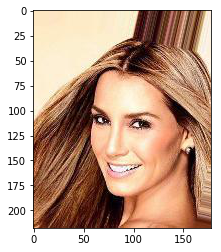
data_loader = batch(filelist)
위까지 하면 batch가 만들어지고.. 한번 뽑아보자!
with tf.Session() as sess:
sess.run(tf.global_variables_initializer())
coord = tf.train.Coordinator()
threads = tf.train.start_queue_runners(sess=sess, coord=coord)
im = sess.run(data_loader)
coord.request_stop()
coord.join(threads)
im = im.astype(np.uint8)
plt.imshow(im[8, :])
<matplotlib.image.AxesImage at 0x7f95ac1a75f8>
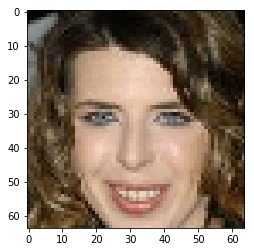
02 Nov 2017
|
ml
generative model
참고 1
생김새
요래 생겼다.
푸는 문제
where
D 관점에서 (1)의 앞 뒤의 수식을 따로 생각해보면
-
- data에서 나온 x(real data)는 값을 크게하고
-
- $p_z$를 통해 나온 $z$가 Generator를 거쳐 나온 G(z)(fake data)는 값을 작게해야
V(D, G)를 크게할 수 있다.
이제 G 관점에서 (1)의 뒷 수식만 보면
-
- 이걸 minimize하려면 $G(z)$가 최대한 x의 분포에 가깝게 가야한다.
논문에 있는 그림인데,
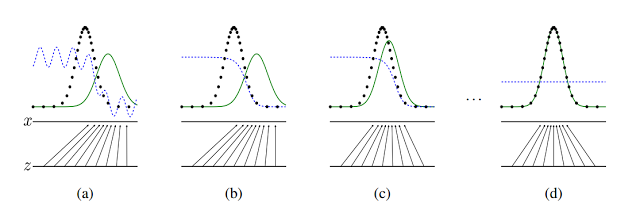
- 검은색: data distribution
- 초록색: generator distribution
- 파란색: discrimimnator distribution
실용적인 수식 변환
사실 (1)에서 $log(1-D(G(z)))$를 minimize하는 대신 $log(D(G(z)))$를 maximize 한다. 처음에 G가 너무 구려서 Discriminator가 구분을 잘함. 그래서 $log(1-D(G(z)))$에 대한 gradient가 0에 가까움.. 근데 $log(D(G(z)))$는 괜찮음.
이론
제안 1.
G가 고정되어있으면 최적의 discriminator D는
증명 >
- 일단 이것부터 증명하자.
$\forall (a, b) \in \textbf{R}^2 - {(0, 0)}$에 대해 어떤 함수 $y \rightarrow a\cdot log(y) + b\cdot log(1-y)$가 존재한다면 [0, 1]사이에서 f(y)가 최대가 되는 y는 $\frac{a}{a+b}$.
미분해서 0이되는 y를 찾으면 당연히 저 값이 나온다. 로그 두개를 하나는 반대로 만들어서 더한 꼴인데, 볼록한 형태일테고… 쉬우니까 넘어가자.
1을 증명했으면 이제 $V(D,G)$를 전개해보자
인데 제안 1을 사용하면 증명 끝이지?
위의 제안으로부터 $ \underset{ D}{max} V(D, G) $는 다음처럼 바뀐다.
Thorem 1.
$C(G)$의 global minimum이 달성 <=> $p_g = p_{data}$. 그리고 그 때 $C(G)$의 값은 $-log(4)$
증명>
- $p_g = p_{data}$일 때 C(G)값 증명
$p_g = p_{data}$면 $D^*_G(x)=\frac{1}{2}$는 당연하고.. 이를 $C(G)$에 넣어보면
- (<=>)증명
이제 KL이나 JSD가 >=0 이니까 최저값은 -log(4)이고 그 때 $p_{data} = p_g$
두번째서 세번째 넘어가는것만 보이자
이제 남은 건 G, D가 충분한 capacity일 때 GAN 알고리즘이 $p_g = p_{data}$를 achieve 하는가 증명인데…. 이건 패스
01 Nov 2017
|
ml
bayesian inference
mcmc
2 Binomial이 독립인 사건에 대해서 analytic하게 풀기
- $\theta_1, \theta_2$가 각각 다른 동전이 앞면이 나올 확률을 의미한다고 가정해보자.
- 그렇다면 likelihood는 다음처럼 정의된다.
- $p(D\vert \theta_1, \theta_2) = \theta_1^{z_1}(1-\theta_1)^{(N_1-z_1)}\cdot\theta_2^{z_2}(1-\theta_2)^{(N_2-z_2)}$
- 또한 posterior는
- $p(\theta_1, \theta_2 \vert D) = p(D\vert \theta_1, \theta_2)p(\theta_1, \theta_2)/ \left [ \int\int p(D\vert \theta_1, \theta_2)p(\theta_1, \theta_2)d\theta_1d\theta_2 \right ]$
- 요기서 두 $\theta$가 독립이라고 하면 ch 5에서 본 beta distibution을 사용해서 또 analytic하게 풀 수 있다.
-
- 정리하면 가 나온다.
approximation -> Gibbs
- parameter space가 작을 때는 grid approximation이 좋은 대안이다.
- 어떤 prior distribution도 쓸 수 있다.
- 어떤 posterior에서 HDI 구할 때도 편하다.
- 하지만 parameter가 넓을 경우는 힘들어진다.
- 그럼 7장의 Metropolis algorithm을 쓰면?
- proposal dist.의 디자인에 따라서 local region에 계속 머물수도 있다.
- Gibbs sampling을 소개해보자
Gibbs sampling
- proposal distribution같은거 없음!
- 대신 나머지 파라미터가 주어진 posterior dist.에서 sampling이 가능해야함(더 빡셈)
- 즉 $\theta_1, \theta_2, \theta_3$이 parameter라고 하면
- $p(\theta_1\vert \theta_2, \theta_3, D)$
- $p(\theta_2\vert \theta_1, \theta_3, D)$
- $p(\theta_3\vert \theta_1, \theta_2, D)$
- 에서 모두 sampling이 가능해야함.
- 엄청 빡센데, 요건만 만족하면 Metropolis algorithm보다 훨씬 reliable하다.
- 이 놈 역시 MCMC process
- 현재 position에서만 다음 스텝이 결정되고,
- sampling을 통해 simulation하니까
- 보통은 순서를 정해서 파라미터를 순회하면서 sample한다.
- parameter가 많아지면 random하게 파라미터를 뽑을 시, 다 뽑는데 시간이 걸림
- Gibbs sampling은 Metropolis에서 proposal distribution이 지금 뽑을 parameter에 의존하는 것이라고 볼 수 있다고 한다. (이 부분 좀 잘 모르겠다..)
- 이 경우 proposal distribution이 항상 그 파라미터의 posterior probability를 반영해서, proposal이 항상 accept된다.
여기까지 보고 다시 위의 2 Binomial 예제를 Gibbs sampling으로 풀어보자!
사실 위의 예제는 너무 단순하긴한데… 그대신 쉬우니까…
- $\theta_1$에 대한 posterior는
- 요걸 가지고 실험을 해보자!
Gibbs Sampling 예제
동전던지기의 예제를 들어본다. $\theta_n$은 n번째 동전이 앞면이 나올 확률을 의미하는 parameter이며, 동전은 2개이다.
가정
- Prior
- $p(\theta_n): beta(\theta \vert 3,3)$
- Likelihood
- $p(z_n,N_n \vert \theta_n): {\theta_n}^{z_n}(1-{\theta_n})^{(N_n-z_n)}$
- data
- 동전 1 - 7번 던져서 5번 앞면
- 동전 2 - 7번 던져서 2번 앞면
%matplotlib inline
import numpy as np
import matplotlib.pyplot as plt
data = [{'N': 7, 'z': 5},
{'N': 7, 'z': 2}]
def posterior(N, z, a, b):
return np.random.beta(z + a,
N - z + b)
thetas = [[0.2, 0.5]]
for i in range(100000):
idx = i % 2
theta = thetas[-1].copy()
theta[idx] = posterior(data[idx]['N'],
data[idx]['z'],
3,
3) # prior가 좀 세다. 1로 바꾸면 좀 더 data와 비슷하게 나옴을 알 수있다.
thetas.append(theta)
thetas = thetas[int(len(thetas)*0.1):] #burn-in
np_thetas = np.array(thetas)
x = np_thetas[:,0]
y = np_thetas[:,1]
plt.plot(x[:300], y[:300], '-o')
plt.show()
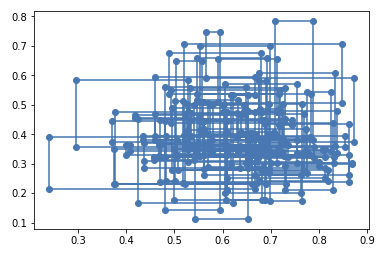
from mpl_toolkits.mplot3d import Axes3D
import matplotlib.pyplot as plt
fig = plt.figure()
ax = fig.add_subplot(111, projection='3d')
hist, xedges, yedges = np.histogram2d(x, y, bins=20, range=[[0, 1], [0, 1]])
xedges, yedges = np.meshgrid(xedges, yedges)
surf = ax.plot_wireframe(xedges[:-1,:-1],
yedges[:-1,:-1],
hist,
linewidth=1)
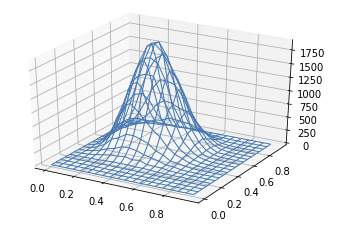
Gibbs sampling의 단점
- 각 파라미터의 conditional probability가 필요!
- 연관도가 높은 파라미터들에 대해서 progress가 멈출 수 있음
$\theta_1$과 $\theta_2$가 Highly correlated 면 왼쪽과 같은 그림이 되겠다. 이 때, 파라미터 업데이트는 오른쪽처럼 아주 작게 움직이겠다.